Karl Oskar takes over Work Package 5
Hello! I'm a third-year pathology resident, working at the department of pathology, Stavanger University Hospital.
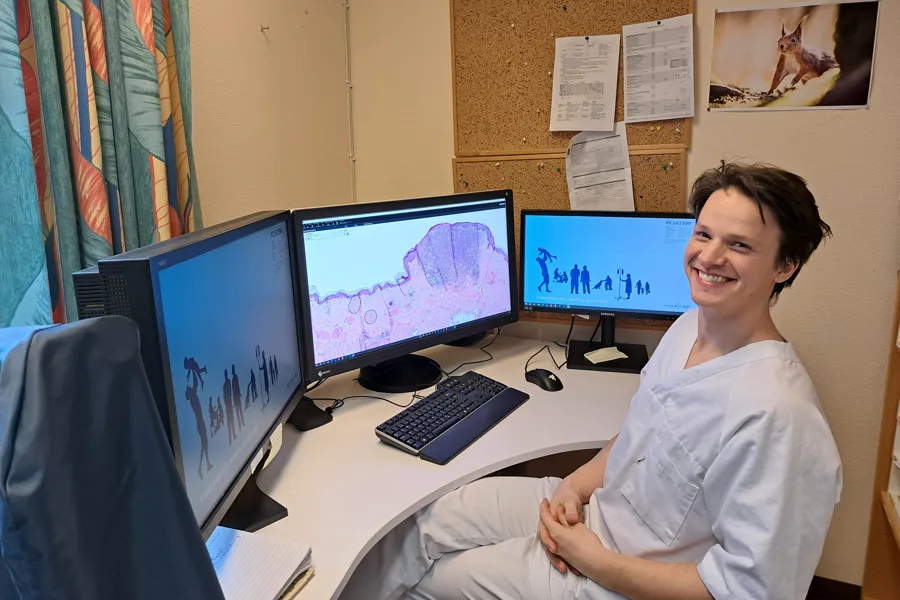
I completed medical school in Bergen in 2018, and after finishing my internship at Helgeland Sykehus, I started working at the department of pathology at Haukeland University Hospital in 2020. I then moved to Stavanger with my family in 2022. I was drawn to pathology because I enjoy visual diagnostics and a more contemplative workday, where pathology is a perfect fit.
I was lucky enough to have experienced the shift from analog to digital pathology firsthand. Building on the positive experiences I’ve had with digitization, I want to be a part of the process of innovation and improvement in the field.
So, in 2023, I was given the opportunity to join the group and the work that has been done in Work Package 5: Automatic Classification of Skin Lesions. In this Work Package we’re developing a Computer-aided Diagnostic (CAD) system for whole slide images, looking at melanocytic lesions. By developing an artificial intelligence model that classifies lesions as benign, unknown, and malignant, we can use the data to develop a diagnostic pipeline: We can prioritize samples by risk for malignancy, making sure high-risk samples are seen early by the pathologist, as well as highlighting regions of interest (ROI) in the actual slide and reducing diagnostic variation. Furthermore, we’re developing machine learning algorithms to predict prognosis in cases of malignant melanoma and implementing molecular data to refine both diagnostics and predictions.
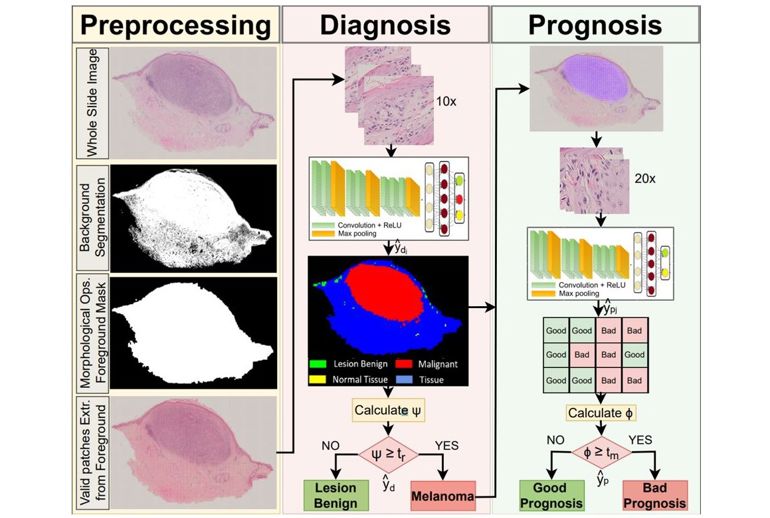
Together with master’s students at Stavanger University (UiS), we’re labeling slides and training an algorithm to recognize the different features that are being used in daily practice to differentiate between malignant and benign lesions. So far, the work has been highly supervised, where pathologists use their domain knowledge to label the different areas of the slide into different types of normal tissue, inflammatory cells, and malignant tissue. These data are processed in an AI pipeline, producing the aforementioned diagnostic classification, ROI's, and prognosis.
I’m picking up the work that has been done by Helga Hardardottir and co-workers, who have developed a system for diagnostics and prediction. Our future challenges lie in increasing the amount of data accessible through different methods of labeling, thus making the already proposed algorithms more robust.